Top 5 AI Ethical Issues that Can Impact Your Research Integrity


In a recent blog, we discussed responsible AI in research and why it matters. Now, we’ll discuss some AI ethical issues and what you should not be doing with AI in your research journey. This blog looks at common mistakes people make with AI in research, explains why they happen, and offers practical tips to avoid them.
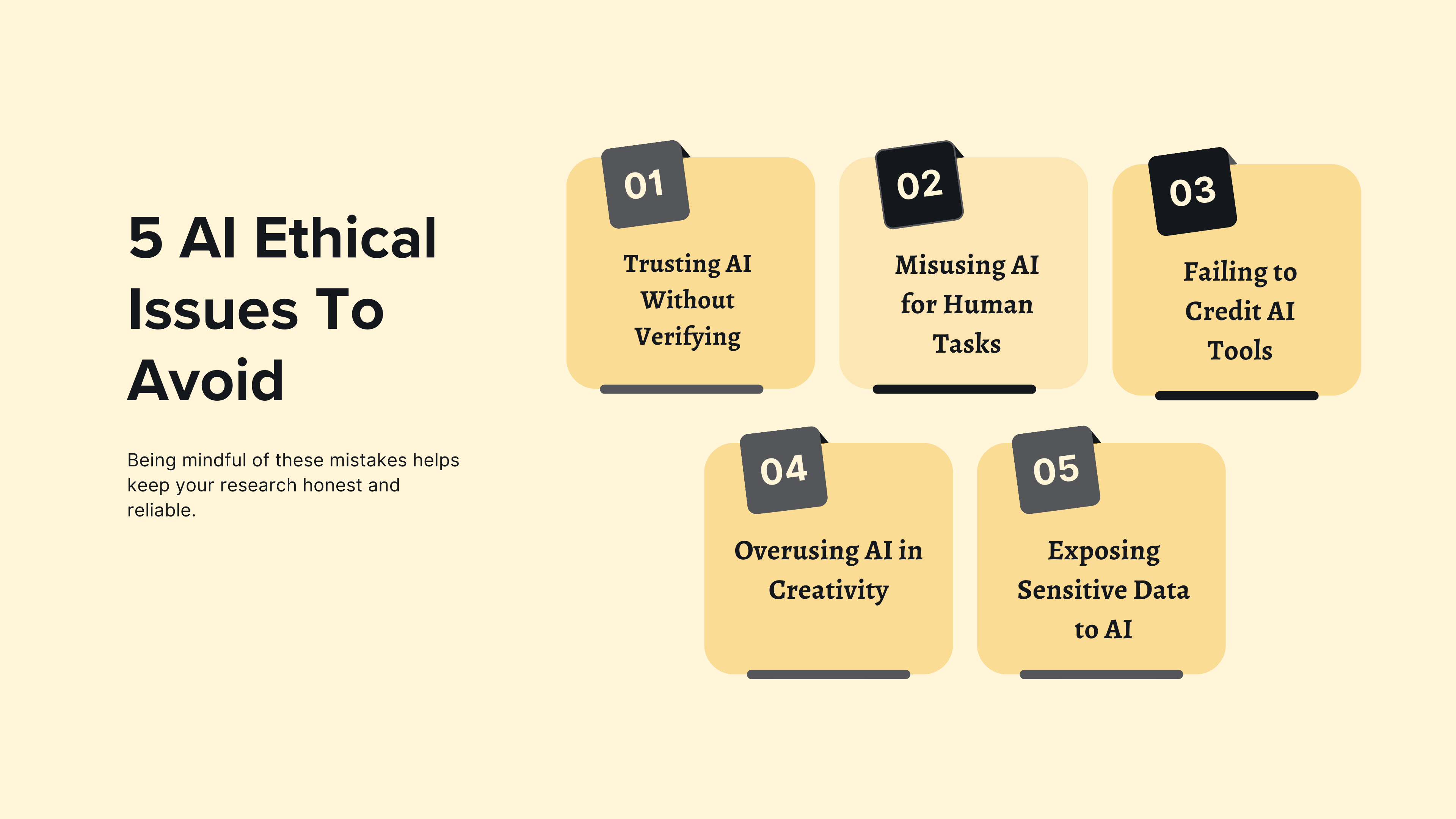
1. Trusting AI Outputs Without Checking Them
One big AI ethical issue is trusting everything AI tools generate without taking the time to verify it. AI models like ChatGPT can produce convincing answers, but they’re not always accurate. In research, this can lead to spreading incorrect information or drawing the wrong conclusions.
Why It Happens: AI systems learn from existing data, which might include errors or biases. As a result, they can unintentionally repeat those issues.
What You Can Do: Treat AI-generated content as a helpful draft, not the final word. Always double-check the information with reliable sources.
2. Using AI for Tasks That Require Human Judgment
Relying on AI for decisions that need a human touch, like reviewing academic papers, is risky. These tasks often require context and empathy, which AI doesn’t have.
Why It Happens: AI seems efficient, but it doesn’t understand the subtleties of human situations, leading to potential AI ethical issues in judgment and fairness.
What You Can Do: Let AI assist with organizing or summarizing information, but make sure a person is involved in decisions that affect others.
3. Not Giving Credit to AI Tools
Even when AI is used responsibly, failing to acknowledge its role can mislead readers about the originality of your work.
Why It Happens: People might not think of AI as a source that needs to be cited, overlooking important AI ethical issues related to transparency and attribution.
What You Can Do: Treat AI tools like any other resource. Check your institution’s or publisher’s guidelines for how to cite them properly.
4. Over-Reliance on AI for Creative Thinking
AI can handle repetitive tasks, but depending on it too much can stifle human creativity. Research often involves brainstorming new ideas, which AI can’t do as well as people.
Why It Happens: AI makes routine tasks more manageable, so letting it take over more complex ones is tempting.
What You Can Do: Use AI to free up critical thinking and creative problem-solving time. Let it handle the busy work while you focus on the bigger picture to avoid these AI ethical issues.
5. Giving AI Access to Sensitive Data
Allowing AI tools to access personal information without proper permission can pose serious security risks.
Why It Happens: Some AI tools require access to data to function effectively, but their security measures might not be sufficient leading to potential AI ethical issues.
What You Can Do: Limit the data AI tools can access. Use platforms with strong security features and comply with data protection regulations.
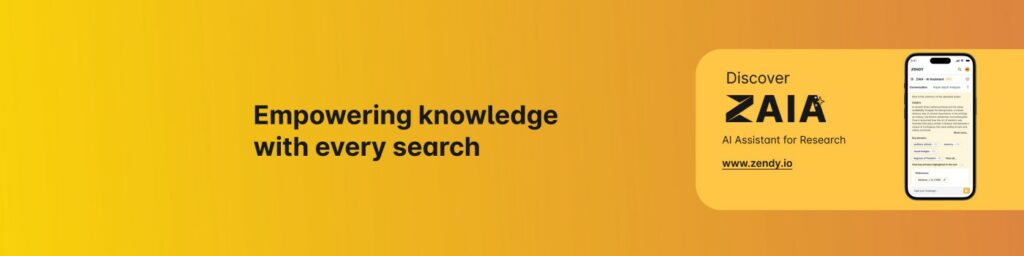
Final Thoughts
AI can be a valuable tool for researchers, but it’s not without its challenges. Many of these challenges stem from AI ethical issues that arise when AI is misused or misunderstood. By understanding these common mistakes and taking steps to address them, you can use AI responsibly and effectively. The key is to see AI as an assistant that complements human effort, not a replacement.
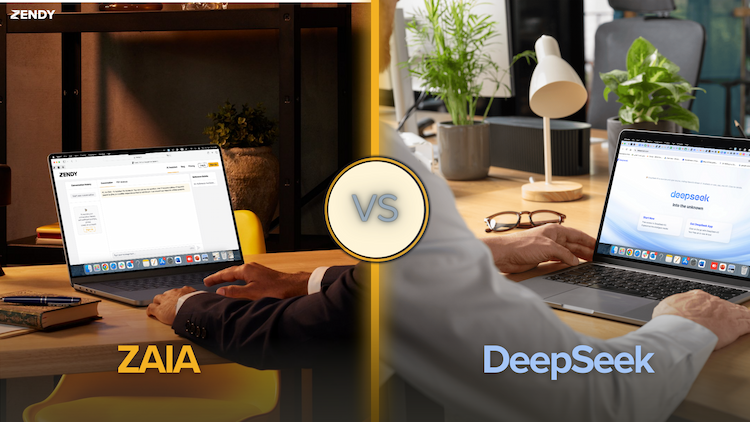
ZAIA vs DeepSeek: Which AI Tool is Better For Researchers
AI tools have made handling complex tasks easier, but not all of them are created equal. While DeepSeek is widely known and accessible, it is a general-purpose AI tool, available to the public and easily understood by many. In contrast, ZAIA, an AI assistant for researchers developed by Zendy, takes a more specialised approach. Designed specifically for researchers and academics, ZAIA easily integrates into academic workflows with customised tools. Let’s take a closer look at how these two AI tools compare. What is DeepSeek DeepSeek AI is a Chinese conversational AI chat created by Liang Wenfeng, mostly used as a general-purpose AI tool that can assist with writing, brainstorming, coding, and more. It’s a Swiss Army knife, good for a lot of things but not customised to a specific need. This makes it a great option for users who need an all-in-one AI assistant rather than a highly specialised tool. What is ZAIA ZAIA, Zendy’s AI assistant, is built with researchers in mind. You can ask any research-related question, and ZAIA will analyse millions of academic papers to provide credible, reference-backed answers. In addition, It’s integrated into Zendy to give you access to over 40 million academic research papers and offers AI tools that make chatting, summarising, analysing, and extracting insights from research papers much simpler. ZAIA and DeepSeek Data & Knowledge Sources One of the key differences between ZAIA and DeepSeek is how they access and process information. ZAIA is designed by researchers for researchers, providing direct access to a vast collection of 40 million scholarly publications through Zendy’s massive digital library. This means researchers retrieve precise insights for their research, summarise it, and chat with it, all from credible, peer-reviewed sources. DeepSeek, on the other hand, is trained on a broad dataset covering various topics but lacks integration with academic databases like Zendy, PubMed or even SpringerLink, and cannot verify sources with citations. While it can generate general information, it lacks the ability to pull insights directly from academic literature, with a high possibility of predatory journals in the outputs. So, what does DeepSeek do better? As we mentioned above, it helps you in answering questions, brainstorming ideas, helping with writing, summarising information, and providing explanations on complex topics in a clear, understandable way. A Smarter, Lower Energy Consumption Choice Since AI became available to the public, concerns about energy consumption have begun to rise. For instance, DeepSeek-V3 required 2,788,000 GPU hours for training, equivalent to 836,400 kWh of energy. According to NDTV News, this consumes less energy compared to the current leading U.S. AI model, ChatGPT. According to RW Digital, the annual energy consumption for ChatGPT is expected to hit 226.8 GWh. To give you an idea of how much energy that is: It could fully charge 3.13 million electric vehicles, which is nearly 95% of all electric vehicles in the United States. It could power around 21,602 U.S. homes for a whole year. It would be enough to run the entire countries of Finland or Belgium for one day. On the other hand, ZAIA was developed to minimise energy usage even more, consuming as little as several kilowatts (kW) per server in data centres ZAIA’s focus on academic tasks means it requires fewer resources. This makes it an environmentally friendly choice for researchers who value sustainability. Who Should Use Which? If you’re looking for a general-purpose assistant to handle a variety of tasks, DeepSeek is a good option. But if you’re a student, researcher, or academic professional who works with research papers regularly, ZAIA is a better fit. It’s built to simplify the research process and provide you with the necessary AI tools and access to your academic needs, integrating AI in scientific research for enhanced productivity and quicker discoveries. Conclusion DeepSeek is a great all-around tool, but ZAIA’s focus on the academic field makes it the smarter choice for researchers. It’s affordable, consumes less energy and time, and is designed to make your academic journey smoother. That’s why, when it comes to AI tools, personalisation is the key. .wp-block-image img { max-width: 85% !important; margin-left: auto !important; margin-right: auto !important; }
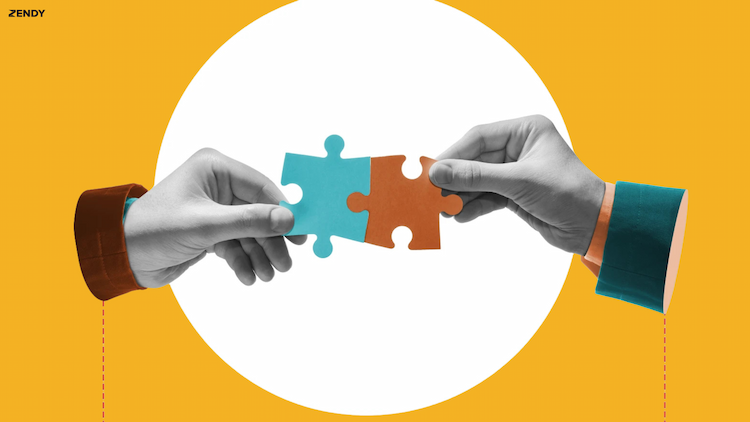
What is Synthesis in Research? Synthesis vs. Summerising vs. Analysis
Going through mountains of papers, studies, and data is a common task when working on a research project. Finding a logical approach to combine all of this information is the hard part. This process is called synthesis, a skill that can help make your research be clearer, more insightful, and more impactful. In simple terms, synthesis in research means combining ideas from different sources to create a new understanding. But here’s the thing: synthesis is often confused with summarising or analysing, which can lead to unclear or incomplete results. In this blog, we’ll break down what synthesis really means, how it’s different from other processes, and how you can do it well. Let’s get started. What is Synthesis in Research? It's about connecting ideas, data, and findings from multiple sources to generate a new perspective. It’s not just repeating what others have said—it’s about connecting the dots to see the bigger picture and how sources relate to your main idea For instance, suppose you are researching the effects of exercise on mental health. One study may indicate that yoga enhances mood, another suggests jogging lowers anxiety and a third that emphasises the advantages of team sports for social well-being. In order to conclude that different kinds of exercise have different positive effects on mental health, synthesis in research involves connecting these studies together. How is Synthesis in Research Different from Summarising and Analysing? A lot of researchers mix up synthesis with summarising or analysing. Here’s how they’re different: Synthesis vs. Summarising Summarising Summarising means condensing the key points of a source or multiple sources without adding new insights. Example: If three studies say that exercise improves mental health, a summary would list those findings without connecting them. Synthesis goes a step further. It combines ideas from multiple sources to create a new understanding. Example: Connecting findings about running, yoga, and team sports to show how different types of exercise benefit mental health in unique ways. Synthesis vs. Analysis Analysis involves breaking down a single source or idea to examine its parts, strengths, or weaknesses. It’s about looking closely at one piece of information. Example: Analysing a study on exercise and mental health might involve critiquing its methods or interpreting its results in detail. Synthesis does not just connect ideas but constructs a new argument or framework from them. It’s about seeing how different pieces of information relate to each other. Example: Synthesising multiple studies on exercise and mental health might reveal patterns, like how different types of exercise affect different aspects of mental health. Why Is Synthesis in Research Important? Because it helps you: See Connections: By combining insights, you can understand a topic more fully. Find Gaps: It helps you spot what’s missing in the existing research. Build Stronger Arguments: When you connect ideas from multiple sources, your conclusions become more convincing. Make Research Useful: In fields like policy or healthcare, synthesis helps turn research into practical solutions. Without synthesis, research can feel scattered or repetitive. It’s what ties everything together. How to Do Synthesis in Research: A Step-by-Step Guide Here’s a simple way to approach synthesis in research: 1. Start with a Clear Question Before diving into your sources, ask yourself: What am I trying to learn or answer? A clear question will help you stay focused and avoid getting overwhelmed. 2. Gather Your Sources Look for reliable, relevant studies, articles, and data. Use tools like Zendy to find the latest articles in one place and EndNote to keep everything organised. 3. Look for Patterns and Connections As you read, take notes on common themes, differences, or trends. For example, do multiple studies point to the same conclusion? Are there conflicting results? A table or chart can help you visualise these patterns. 4. Bring It All Together Combine the insights you’ve gathered into a cohesive narrative. Explain how the ideas relate to each other and to your research question. 5. Draw Your Conclusions Summarise what you’ve learned and explain why it matters. Be honest about any limitations and suggest areas for further study. How can you improve your synthesis skills? Read your sources multiple times. Make well-organised notes on each one. Find relevant ideas and evidence for them. Rearrange your notes based on concepts. Put ideas into an outline. Conclusion Synthesis in research is more than just combining information—it’s about creating new knowledge and understanding. By following the steps above, you can effectively bring together ideas, spot patterns, and produce work that’s clear, insightful, and useful. Whether you're a student, academic, or professional, synthesis can help you take your research to the next level. Struggling to make sense of your research? AI tools can assist with synthesis by identifying key themes and connections across multiple papers, check out our AI tools to simplify the process and get better results. Let’s make your research work for you! .wp-block-image img { max-width: 85% !important; margin-left: auto !important; margin-right: auto !important; }
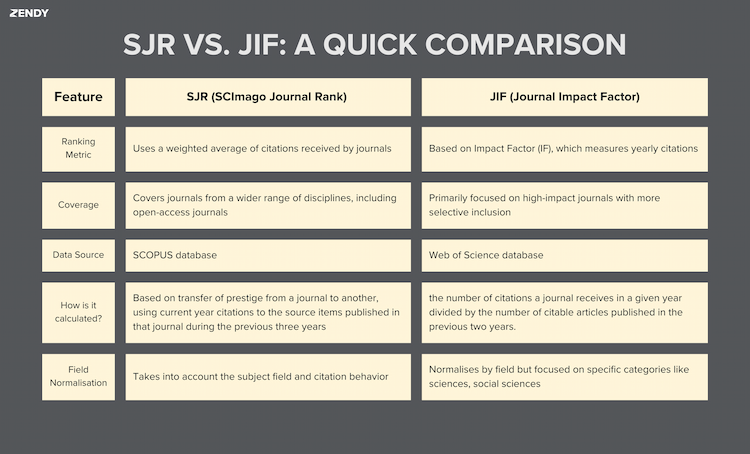
JIF vs. SJR Ranking: What's The Difference?
Two of the most common journal rankings are the Journal Impact Factor (JIF) and SCImago Journal Rank (SJR). They both measure journal impact but in different ways. Knowing how they work can help you choose the right journal to publish in. If you're deciding where to publish your research or assessing the significance of journals in your field, understanding these metrics can guide your choices. While both JIF and SJR aim to measure journal impact, they approach it differently. Depending on your needs, you might prefer one over the other or consider both for a well-rounded view. What Is SJR? Developed by SCImago using Scopus's database, SJR ranking evaluates the scientific impact of journals on their citations. Unlike a simple citation count, SJR assigns a higher value to citations from prestigious journals, meaning that not all citations carry the same weight. Key Features of SJR Ranking: Citation Quality Is Important: A journal's SJR ranking is influenced more by citations from reputable publications. Three-Year Citation Window: Citations are taken into account by SJR ranking for a duration of three years. Field-Normalised: SJR ranking takes into consideration variations among disciplines, which facilitates the comparison of publications from various fields of study. Open Access Inclusion: By incorporating open-access journals, SJR ranking provides a more comprehensive understanding of journal impact. What Is JIF? The Journal Impact Factor (JIF) provides one of the most well-known indicators of a journal, run by Clarivate and based on Web of Science database. Unlike SJR ranking, JIF is calculated by a straightforward ratio: the number of citations a journal receives in a given year divided by the number of citable articles published in the previous two years. Key Features of JIF: Two-Year Citation Window – Focuses on recent citations. Straightforward Calculation – It is simple to understand because it uses a simple average. Exclusive to Web of Science – Only Web of Science-indexed journals are included. Heavily Used in Academic Promotion – JIF is often a deciding factor in funding applications and tenure evaluations. Which Ranking Should You Use? It depends on what you're looking for. If you need a broader view of journal impact that considers citation prestige, SJR ranking may be more useful. If you're in a field where the Journal Impact Factor is commonly used for assessments (e.g., life sciences, medicine), JIF might be the better choice. If you're working with open-access journals, SJR provides a more inclusive perspective. In Conclusion While each ranking system has advantages, none of them is a perfect measurement of journal ranking quality. To have a better view of a journal's influence, think about examining a variety of classifications rather than depending solely on SJR ranking or JIF ranking. Knowing these distinctions will help you make well-informed choices, whether choosing a journal for publication or assessing a research paper. Sources: Clarivate Analytics Impact Factor SCImago Journal Rank (SJR) .wp-block-image img { max-width: 85% !important; margin-left: auto !important; margin-right: auto !important; }