AI is Transforming Academic Research and Publishing – A Conversation with Kamran Kardan, CEO of Zendy


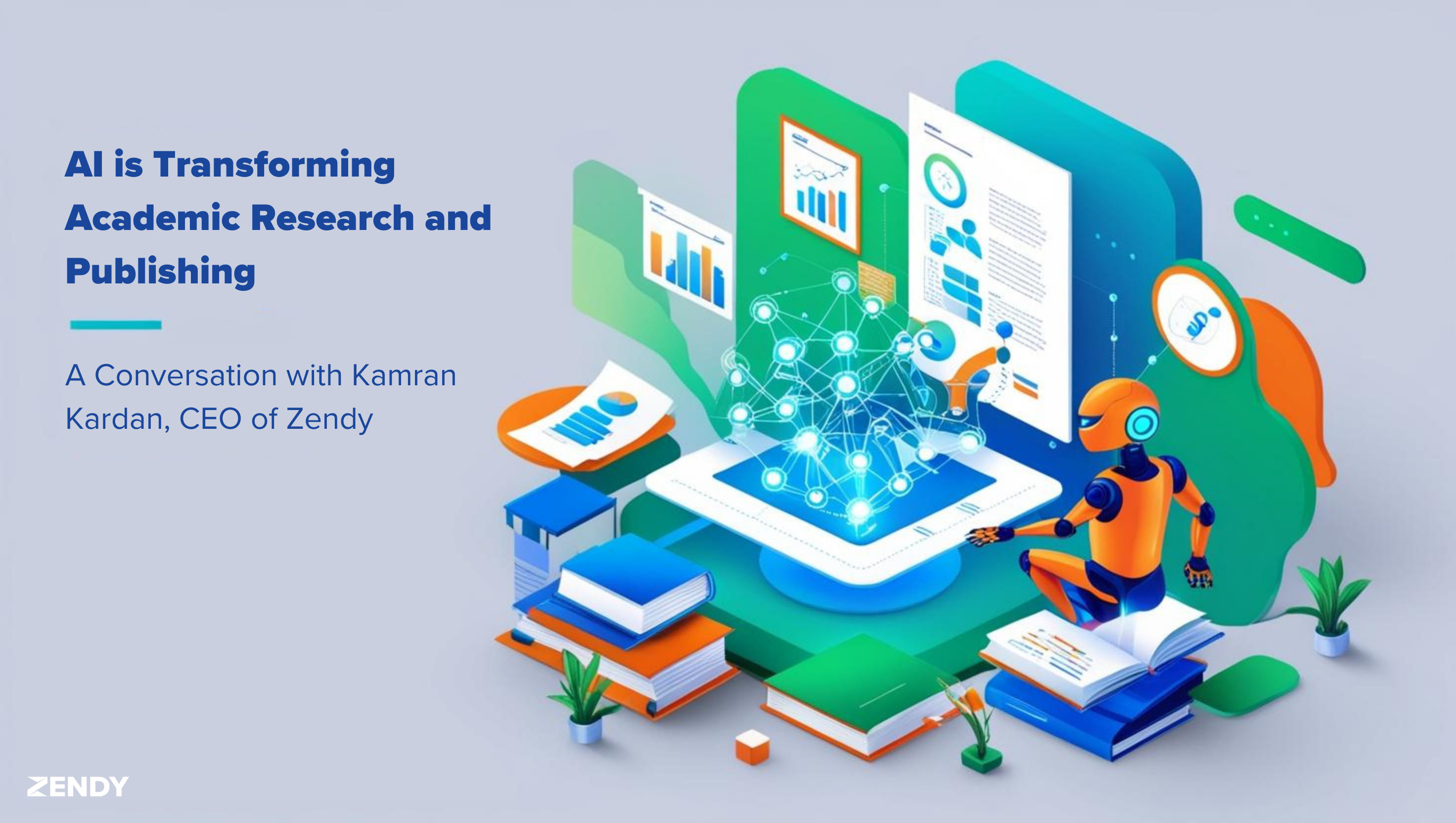
AI's real potential lies not just in speeding up processes but also in helping users engage more deeply with academic content. Sabine Louët, CEO of SciencePOD sat down with Kamran Kardan, CEO of Zendy to discuss how technology, particularly AI, is reshaping the way researchers and independent scholars access critical information and how research is published.
Removing Barriers in Academic Research
When asked by Sabine Louët “what drove the creation of Zendy?” Kamran Kardan’s response was clear and purposeful: “Zendy was created to remove the barriers that restrict access to academic research”.
He highlights the significant gap that exists for those outside privileged institutions, who often face prohibitive costs or limitations when trying to access essential research. Zendy, he says, aims to make academic content not only affordable but also widely accessible to researchers, students, and professionals globally.
Accessing scientific literature remains a privilege reserved for those with institutional affiliations, leaving independent researchers or those from less-resourced regions at a disadvantage. As Kardan puts it, “Zendy is committed to levelling the playing field”, it offers a legitimate alternative to illicit means of accessing research.
AI’s Role in Enhancing Research Accessibility
AI has become a buzzword, but Kardan stresses the importance of AI in Zendy’s strategy, describing AI as an enabler rather than the focal point. Zendy, he explains, uses AI to enhance user experience by making vast amounts of data more navigable.
One of the platform’s key AI-driven features is its summarisation tool, which allows users to quickly digest complex academic papers. With this tool, users can identify relevant content faster and focus their research efforts more effectively.
A forthcoming feature called ‘findings’, will use AI to group related articles together, offering a comparative perspective on topics and highlighting differing viewpoints. This tool is designed to empower researchers to explore a topic from multiple angles without having to sift through unrelated material.
Safeguarding Research Integrity in the Age of AI
Another point of discussion between Sabine Louët and Kardan was the issue of integrity while also leveraging AI. Kardan acknowledges that this is critically important and explains that Zendy is built on principles of transparency and respect for intellectual property. Their AI tools do not merely extract data but give due credit to authors and publishers.
In addition, the platform’s revenue-sharing model ensures that content creators benefit from the usage of their work, fostering a more sustainable and fair ecosystem for academic publishing.
Kardan also addresses the issue of AI-generated inaccuracies, commonly referred to as “hallucinations”. He emphasises that Zendy's AI is structured to avoid these risks. If the AI does not have sufficient data to provide an answer, it refrains from making assumptions, thus maintaining a high standard of accuracy.
AI: Not Just Speed, but Deeper Learning
In Kardan’s view, AI's real potential lies not just in speeding up processes but also in helping users engage more deeply with academic content. The tools developed by Zendy are designed to simplify complex materials, making them more approachable for users across various disciplines, without compromising on the depth of information.
Louët agrees and notes that these features, particularly AI-driven comparison and summarising tools, align with the needs of modern researchers who require both efficiency and reliability in handling academic content.
Looking Ahead: The Future of AI in Research
What does the future look like? Kardan foresees more AI advancements that will continue to transform research access, making it more affordable, transparent and equitable. The focus is not just on technology for technology’s sake but on providing meaningful solutions that directly address the challenges of the academic community.
“AI’s role in academic publishing is still evolving”, says Kardan, “and Zendy is committed to using AI responsibly to enhance access to knowledge, not to replace human expertise”.
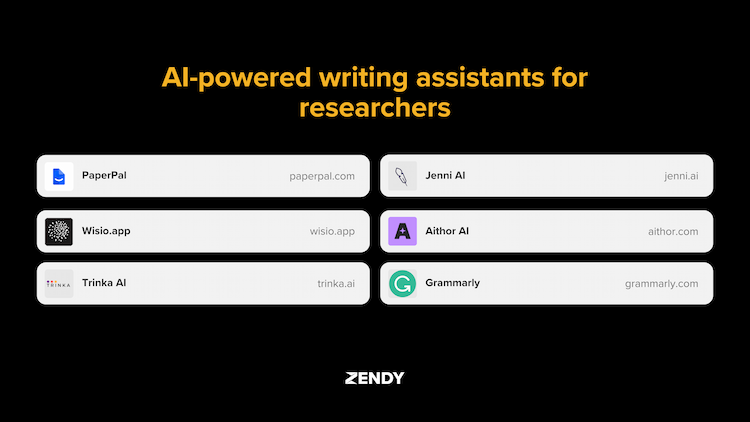
Top 6 AI Writing Assistant Tools for Research
Many students and researchers today use artificial intelligence (AI) to help improve their writing. These tools are not only for checking spelling or grammar, but they can help organise ideas, improve sentence structure, and manage citations. Writers working on research papers often spend extra time editing and citing sources correctly. AI writing assistant tools are designed to support those specific tasks by using advanced language technology. In this article, we explore how AI writing assistant tools like PaperPal, Jenny.AI, Aithor, Wisio.app, Trinka AI, and Grammarly work. Each tool offers a different approach to writing assistance, depending on what kind of research you are doing and what stage you are in. What are AI Writing Assistant Tools AI Writing Assistant Tools are software applications that utilise artificial intelligence to enhance writing. They analyse text using machine learning and natural language processing (NLP), which allows them to detect issues with grammar, tone, structure, and clarity. Natural language processing is a type of AI that helps computers understand and generate human language. This technology allows writing assistants to do more than just catch spelling errors, they can suggest rewording, offer synonyms, and help improve sentence flow. Early writing tools mainly checked for spelling and punctuation. Over time, they evolved into systems that assist with academic writing, including literature reviews, paper organisation, and citation formatting. Main benefits of AI writing assistant tools: Time Efficiency: These tools speed up writing by suggesting edits and checking grammar in real time. Language Enhancement: They improve sentence structure and formal tone for academic audiences. Citation Management: Many tools generate citations and apply citation styles automatically. Research Workflow: Some AI writing assistant tool helps structure research papers by suggesting outlines. Comparing Key Research Writing Assistants The table below compares six AI writing assistant tools used in academic research: Tool NameBest ForKey FeaturesFree VersionPaperPalJournal submissionsJournal formatting, grammar checksYesJenny.AIDrafting academic contentAI autocomplete, citation generatorYesAithorStructured draftingPlagiarism detection, writing suggestionsYesWisio.appPeer-reviewed feedbackHuman and AI editing, multilingual supportLimitedTrinka AIESL academic writingTechnical term support, citation formattingYesGrammarlyGeneral writingGrammar checks, browser integrationYes Language Enhancement Capabilities Each tool approaches grammar, tone, and style differently: PaperPal: focuses on academic publishing with discipline-specific language suggestions. Jenny.AI: offers real-time assistance through AI autocomplete for academic writing. Aithor: helps users draft content with tone guidance and structure prompts. Wisio.app: provides detailed editorial feedback tailored to scientific writing. Trinka AI: helps non-native English speakers with academic tone corrections. Grammarly: covers general grammar improvements but adapts to academic contexts. Research Focused Features These tools support research writing in different ways: PaperPal: supports journal-specific formatting and citation checks. Jenny.AI: generates in-text citations and formats reference lists. Aithor: detects unoriginal content and suggests better source integration. Wisio.app: allows collaborative editing with structured feedback. Trinka AI: identifies missing citations and formats according to style guides. Grammarly: includes basic citation suggestions and plagiarism detection. PaperPal PaperPal is an AI writing assistant tool that mostly focuses on helping researchers prepare academic manuscripts. It is designed to support you with the process of submitting papers to journals by ensuring that writing meets formatting and language requirements. The tool includes journal-specific formatting options. This allows researchers and students to format their papers according to the guidelines of a selected journal, including structure, citations, and reference styles. It also provides language support for technical writing by identifying discipline-specific terminology and suggesting corrections to align with academic tone and clarity. Key features: Journal Compatibility: Matches manuscript formatting to journal guidelines, including citation style. Technical Language Support: Refines field-specific vocabulary and academic phrases. Integration Capabilities: Connects with research tools like Overleaf and Word. Jenni AI Jenni AI helps with research-based writing tasks. It drafts academic content, manages citations, and supports the structure of academic arguments. The platform generates text based on prompts or uploaded documents. It works with academic papers and uses AI to build sections of content that align with your topic. Jenni AI also includes citation tools that format references in over 1,700 styles. You can save sources in a library and insert citations directly into your draft while writing. Key features: AI-Powered Drafting: Generates academic content from prompts or uploaded research. Citation Integration: Supports in-text citations and reference management in multiple formats. Collaborative Features: Enables group access to shared libraries and drafts. Aithor Aithor supports the academic writing process while helping maintain originality and proper writing practices. It checks for unoriginal content by comparing written text against existing sources. This helps users revise their work to reduce overlap and avoid academic misconduct. The platform allows users to add scholarly sources into their documents with an interface for inserting citations and generating references using common academic styles. Key features: Original Content Generation: enhances your writing without compromising your originality Academic Integrity Tools: Flags duplicated phrases and offers paraphrasing suggestions. Research Integration: Adds peer-reviewed sources and formats them according to guidelines. Wisio App Wisio supports academic collaboration by helping researchers work together on documents and improve their work through structured feedback. The platform includes systems for reviewers to leave targeted comments on drafts. These comments are organised to help writers identify issues with clarity, logic, or formatting. It also includes tools for managing research projects with task assignments, progress tracking, and draft organisation. Multiple users can edit documents at the same time, seeing changes in real time. Key features: Feedback System: Enables structured peer feedback with in-line comments. Workflow Management: Supports task tracking and drafting stages for collaborative projects. Collaborative Editing: Allows multiple users to edit a document simultaneously. Trinka AI Trinka AI supports writers who speak English as a second language (ESL). Its tools identify grammar and usage issues common among non-native speakers. The platform recognises technical language from various academic fields such as engineering, medicine, and social sciences. It suggests corrections based on the context of the discipline. Trinka also supports researchers preparing manuscripts for publication by checking for consistency with international journal standards, including formatting and language clarity. Key features: ESL Support: Offers grammar correction and formal language suggestions for non-native English writers. Technical Terminology: Refines field-specific vocabulary across multiple disciplines. Publication Standards: Evaluates manuscripts for compliance with journal requirements. Grammarly Grammarly helps users write with correct grammar, punctuation, and clarity. It works in academic, business, and casual writing by scanning text for errors and offering real-time suggestions. For academic writing, Grammarly supports clarity and formal tone by identifying passive voice, informal phrasing, and awkward sentence structure. However, it does not provide research-specific features like citation formatting. The tool works across emails, web browsers, word processors, and mobile apps. While helpful for basic academic editing, its focus is on general writing improvement rather than specialised research tasks. Key features: Universal Applications: Functions in Word, Google Docs, emails, and browsers. Tone Adjustments: Offers suggestions to align writing with academic formality. Integration Ecosystem: Works with Chrome, Microsoft Office, and email clients. How to Choose the Right AI Writing Assistant for Your Research Selecting an AI writing assistant depends on your specific academic task. Different tools support different aspects of the writing process. Evaluating Your Writing Goals Consider what you're writing before choosing a tool: For a thesis, look for long-form structuring and reference tracking. For journal articles, check for journal-specific formatting and academic tone adjustments. For grant proposals, find tools with outlining and collaborative editing features. Some tools help generate initial drafts, while others focus on editing, formatting, and feedback. Integrating AI With Existing Tools AI writing assistant tools work best when they connect with other research tools. Check if the assistant works with reference managers like Zotero or EndNote to maintain accurate citations. Many platforms integrate with word processors like Google Docs, Microsoft Word, or Overleaf. Others allow importing and exporting in formats such as .docx, PDF, or LaTeX. Ensuring Academic Integrity Using AI writing assistant tools raises questions about originality. These tools don't replace human thinking but assist with language and formatting. To use AI ethically: Disclose AI use when required by your institution. Review all AI-generated content manually for accuracy. Revise AI-generated text before submission. Empowering Research Writing and Next Steps AI writing assistant tools have changed how academic writing is planned and processed. These tools help with grammar correction, citation formatting, and research workflow. In the future, AI writing assistant tools will likely offer deeper integration with citation managers, research databases, and publishing platforms. Some may add voice input, multilingual support, and automatic journal formatting. Access to reliable academic sources remains essential for these tools to function effectively. Platforms that provide full-text academic content allow AI writing assistant tools to generate accurate citations and summaries. Zendy offers one such environment by combining scholarly content with AI tools that support literature review and citation. Discover how Zendy's AI-powered research library can enhance your writing workflow at Zendy.io. How do AI writing assistant tools maintain academic integrity? AI writing assistant tools do not generate original research or ideas. They improve grammar, structure, and clarity, allowing the writer's own thoughts and arguments to remain central. Which AI writing assistant offers the best citation management? PaperPal and Trinka AI include built-in tools for formatting citations in academic styles. Jenni AI supports over 1,700 citation formats and allows integration with reference managers. Are free versions of these AI writing assistant tools sufficient for research? Free versions include basic grammar checks but typically exclude advanced features like formatting, citation tools, or deep academic editing. Paid versions provide more comprehensive research support. Can these tools help with discipline-specific terminology? Trinka AI and PaperPal recognise subject-specific vocabulary in fields like medicine, engineering, and social sciences. They check for accuracy and consistency in technical language. .wp-block-image img { max-width: 85% !important; margin-left: auto !important; margin-right: auto !important; }
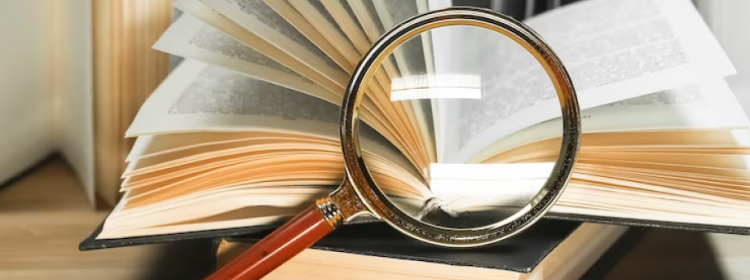
Making Scholarly Research Accessible for Independent Researchers in 2025
Many researchers work outside of universities or formal institutions. These independent researchers often rely on public access to scholarly research to study, write, or contribute to their fields. However, access to scholarly research is not equal. Most academic journals are behind paywalls, which means users must pay to read them unless they are affiliated with an institution that pays for access. In this blog, we’ll explore the structure of academic publishing and how it affects independent researchers. We’ll break down the current challenges, the systems in place, and recent developments designed to improve research accessibility. Why Research Accessibility Matters Research accessibility refers to how easily someone can read, use, and build upon academic studies. For independent researchers, access is often limited because they lack university or library credentials required to unlock paywalled content. A large portion of scholarly research remains behind subscription paywalls. Many journal articles cost between $30 and $50 each, and full journal subscriptions can reach thousands of dollars per year. These costs create a divide between researchers affiliated with institutions and those working independently. Independent researchers may be excluded from current findings, which restricts their ability to contribute to academic conversations. Without equal access, knowledge development becomes uneven. Some communities and individuals are left out, creating a gap in who can participate in scientific and scholarly work. Understanding Open Access Models Open access (OA) refers to academic research that anyone can read online without paying. There are different types of open access, and each works in a specific way. 1. Gold Open Access to Scholarly Research Gold open access means that the final version of a research article is freely available on the publisher's website. The author or their funder usually pays a fee to make the article open. Researchers can find gold open access content in fully open access journals listed in the Directory of Open Access Journals (DOAJ). These journals allow anyone to read and download the scholarly research directly from the publisher. Reader benefit: Immediate access to the final, formatted version of articles Limitation: Authors often pay fees ranging from $500-$3000 to publish 2. Green Open Access Green open access is when authors share a version of their article in a free online repository. This version may be a preprint (before peer review) or a postprint (after peer review but before journal formatting). Repositories like arXiv.org specialise in many disciplines, and bioRxiv.org for biology, host these papers. These platforms do not require any affiliation to access the content. Reader benefit: Free access to research content, often before formal publication Limitation: The version available might not be the final published version 3. Diamond Open Access Diamond open access journals make articles freely available to read and do not charge authors any fees to publish. Neither readers nor authors pay. One good example of diamond open access is KnE Publishing, an open access publishing service by Knowledge E, provides high-quality publishing services to support the development and advancement of diamond open access journals, with a particular focus on increasing the visibility and accessibility of scholarly research. This model is often supported by academic institutions or non-profit organisations. The Free Journal Network lists many of these journals. Reader benefit: Completely free access with no barriers Author benefit: No publication fees to share research Open Access ModelWho PaysWhere to FindVersion AvailableGoldAuthors/fundersPublisher websitesFinal published versionGreenNo one (usually)RepositoriesPreprint or postprintDiamondInstitutions/grantsPublisher websitesFinal published version Practical Tools For Independent Researchers Independent researchers need affordable ways to find and use scholarly research. Several tools make this process easier. AI Summarisers AI summarisers extract the main points from academic papers. These AI tools help researchers quickly understand if a paper is relevant to their work without reading the entire document. Zendy's AI summarisation tool identifies key findings, methods, and conclusions from scholarly research papers. This saves time when reviewing large amounts of literature. Time-saving: Condenses hours of reading into minutes Comprehension aid: Helps readers understand complex academic language Literature Discovery Tools Discovery tools help researchers find academic papers and locate free versions when available. Google Scholar indexes scholarly research and sometimes links to free versions. Zendy uses AI to recommend relevant papers based on your interests. Browser extensions like Unpaywall and Open Access Button automatically find legal, free versions of paywalled articles. Broader search: Searches across multiple journals and repositories at once Free alternatives: Identifies open access versions of paywalled content Scholarly Research Reference Manager Tools Reference manager tools help organise research papers and create citations. These tools are essential for independent researchers writing their own papers. Zotero is a free, open-source reference manager that saves papers, creates citations, and integrates with word processors. Mendeley offers similar features with some social networking elements. Organisation: Keeps research papers in one searchable library Citation help: Automatically formats citations in different styles Policy Shifts Empowering Independent Scholars Recent policy changes are increasing the amount of research that is freely available to everyone. These changes help independent researchers access more content without institutional subscriptions. Plan S requires that research funded by certain organisations be published with open access. This means more high-quality scholarly research is becoming freely available to read. Many funding agencies now require researchers to share their findings openly. The National Institutes of Health in the US and UK Research and Innovation have policies requiring funded research to be publicly accessible. Authors are also finding ways to keep their rights to share their work. Rights retention strategies allow researchers to post copies of their articles in public repositories even when publishing in traditional journals. The trend toward open science continues to grow. More institutions are adopting policies that make research outputs—including data, software, and educational materials—freely available by default. Ensuring Accessibility For All Researchers Accessibility in scholarly research goes beyond open access. It also means making content usable for people with disabilities and those using different devices or internet connections. Universal Design Principles Universal design makes scholarly research usable by as many people as possible. This includes clear structure, readable text, and compatibility with assistive tools. Well-designed articles use proper headings, include descriptions for images, and create documents that work with screen readers. These features help all users navigate and understand the content more easily. Examples of accessible design in scholarly research: Structured headings that create a logical outline Alternative text for images and diagrams Tables with proper headers and simple layouts PDF files with proper tagging for screen readers Assistive Technology Compatibility Assistive technologies help people with disabilities access digital content. Researchers need to work well with these tools. Screen readers convert text to speech for people who are blind or have low vision. Text enlargement tools and colour contrast adjusters help people with different visual needs. When looking for accessible research content: PDF accessibility: Look for tagged PDFs that work with screen readers HTML versions: Often more accessible than PDFs for assistive technologies Plain text options: Simple format that works with most assistive tools If you need a more accessible version of any scholarly research, you can contact the publisher directly. Many journals now provide alternative formats upon request. New Innovations in Research Access The landscape of scholarly access continues to evolve with new models and technologies making research more available to independent scholars. AI-powered research assistants are changing how people interact with academic literature. These tools can summarise articles, extract key information, and help researchers find connections between papers. Digital libraries like Zendy are creating alternatives to traditional subscription models. With AI assistants like ZAIA (Zendy's AI assistant for researchers), these platforms not only partner with publishers to offer access to both open and paywalled content at affordable rates for individual researchers, but also enhance the research experience through AI support. The future of scholarly research access looks increasingly open and innovative. New technologies and business models continue to break down barriers between knowledge and those who seek it. FAQs about Accessing Scholarly Research How can independent researchers find free academic articles legally? Independent researchers can use open access repositories like PubMed Central and preprint servers like arXiv. Public libraries sometimes offer access to academic databases, and contacting authors directly often results in them sharing their papers. What makes scholarly research accessible to people with disabilities? Accessible scholarly research uses proper document structure with headings, provides alternative text for images, creates tables that screen readers can navigate, and offers formats compatible with assistive technologies. Articles in HTML format are typically more accessible than PDFs, and properly tagged PDFs are more accessible than untagged ones. How do researchers evaluate the quality of open access journals? Researchers can check if an open access journal is listed in the Directory of Open Access Journals (DOAJ), look for clear peer review policies, verify the journal's impact factor, and research the editorial board members. Quality open access journals maintain the same rigorous standards as traditional subscription journals. What AI tool helps independent researchers conduct a literature review? ZAIA, Zendy's AI research assistant, helps independent researchers conduct efficient literature reviews by automatically summarising academic papers, extracting key findings, and identifying connections between related studies. Researchers can also use reference managers like Zotero or Mendeley to organise papers and create citations. Literature mapping tools like VOSviewer help visualise research networks and identify influential papers. For comprehensive literature reviews, ZAIA can recommend relevant papers based on your research interests, saving hours of manual searching across multiple databases. .wp-block-image img { max-width: 85% !important; margin-left: auto !important; margin-right: auto !important; }
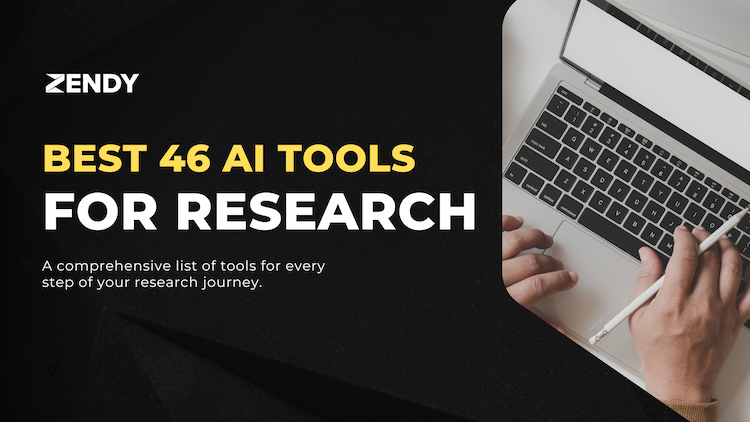
Top 46 AI Tools for Research in 2025 (Writing, Citations, Literature Review & More)
Five years ago, many believed Web 3.0 and a decentralised internet would reshape how we interact online. Instead, the real change came from artificial intelligence (AI). Quietly, it started showing up everywhere, from how we search to how we write and learn. In research, the impact of change is particularly evident. AI tools for research have evolved beyond simple assistance. It's now central to how we study, gather information, and break down complex ideas. A recent 2025 AI survey by Zendy shows just how common AI has become within the research ecosystem: 73.6% of students and researchers say they use AI tools, with over half of them using AI tools for literature reviews and nearly as many using them for writing and editing How We Picked The Best AI-powered Research Tools All AI tools for research listed in this blog were curated based on a combination of personal testing, side-by-side feature comparisons, user feedback across platforms, and their overall visibility and adoption within academic and research circles in 2025. All the AI tools for research were evaluated for their usefulness, ease of use, affordability, and relevance to different stages of the research process, from literature review and note-taking to data analysis, paraphrasing, writing, and presenting. In particular, the AI tools for research that gained traction among students, postgraduates, and working researchers on platforms like Twitter, YouTube, and academic subreddits were prioritised, alongside those with strong academic institution uptake or solid citation in forums and journals discussing AI in education. We also considered tools that had strong community support, frequent updates, and clear pricing models to ensure they are not just powerful, but also practical and sustainable for real-world academic use. In this blog, we’ll walk you through 46 AI tools for research and show you how they can help you with different parts of your workflow. Some are free, some are paid, and many are rapidly evolving in features, pricing, or availability. We’ve done our best to give you an up-to-date overview, but it’s always worth trying a tool yourself to see how it fits into your research process. List of best AI tools for research: AI Research Assistants for Students: ZAIA, Elicit, Perplexity AI, Research Rabbit, Scite, ChatGPT, Connected Papers AI-driven Literature Review Tools:Zendy, Litmaps, ResearchPal, Sourcely, Consensus, R Discovery, Scinapse.io AI-powered Writing Assistants:PaperPal, Jenny.AI, Aithor, Wisio.app, Trinka AI, Grammarly AI Tools for Data Analysis in Research:Julius AI, Vizly, ChatGPT-4o, Polymer, Qlik AI Paraphrasing Tools for Students:Ref-n-write, SciSpace, MyEssayWriter.ai, Scribbr, Rewrite Guru AI Productivity Tools for ResearchersOtter AI, Bit.ai, Todoist, Notion AI Tools for Thesis Writing:TheseAI, Gatsbi, Writefull, Thesify AI Citation Management Tools:Zotero, EndNote, Mendeley, RefWorks AI Tools for Creating Research PresentationsGamma, Presentations.AI, PopAI, AiPPT AI Research Assistants for Students We conducted a recent survey with 1,502 participants through multiple channels to explore AI adoption rates, research habits, and accessibility preferences within academic workflows. The survey, AI for Research: 2025 Trends and Statistics, revealed that 67.6% of respondents who use AI for research are between 18 and 24 years old, reflecting more concentrated AI use across early-career researchers and students, in comparison to more seasoned researchers. Here are some of the favourite AI research assistants for students ZAIA – Zendy’s AI Assistant ZAIA, one of the AI tools for research assistance by Zendy, is designed specifically to help you save time, enhance focus, and summarise complex academic content. Integrated into Zendy’s extensive library of millions of peer-reviewed publications, ZAIA enables fast, credible, and reference-backed answers to research questions. Unlike other AI tools, ZAIA delivers a more targeted and noise-free academic discovery experience by filtering out irrelevant sources to offer researchers laser-focused vision on the topics of research. Best for: Breaking down complex academic language and making research content more accessible. Pros: Saves time by delivering concise, reference-backed insights. Includes a “Chat with PDF” feature for interactive document analysis, tailored for academic and research workflows. Cons: Not suitable for creative writing or technical coding tasks. Elicit Elicit is an AI research assistant developed by Ought that specialises in literature reviews. This tool assists you find and summarise academic papers based on specific questions. It facilitates various research stages like brainstorming, planning, and organising findings. While powerful for early-stage research, it often relies on open-access papers and its database can sometimes lack newer or niche research. Best for: Brainstorming and refining research questions during the early stages of academic work. Pros: Designed specifically for academic workflows; intuitive and question-driven approach. Cons: Limited to open-access sources and does not support full PDF uploads. Perplexity AI Perplexity AI is a conversational AI that acts like a smart search engine, delivering sourced answers across a wide range of topics. It can the information from academic articles, web sources, and news outlets. However, while it provides citations, It is not specifically considered one of the AI tools for research and may reference both scholarly and non-scholarly sources depending on the query Best for: Assisting students in understanding complex subjects. Pros: Available on web, mobile apps, and browser extensions. Cons: Not tailored to academic papers. Research Rabbit Research Rabbit focuses on discovery through visual graphs, helping researchers explore academic papers, authors, and citation networks in a more visual way. It’s especially handy for tracing how a research topic has developed over time and spotting related work. While it's great for that kind of exploration, it doesn’t offer the summarisation features or interactive support you’d find in the other AI tools for research. Best for: Visual mapping of literature. Pros: Integration with Zotero. Cons: Relies on the MAG, which discontinued updating its content in 2021. This means newer publications might not be included in its database. Scite Scite offers a unique twist not just by linking to research papers, but also by showing how papers reference each other, whether positively, negatively, or neutrally. It gives you a clearer picture of the quality and context behind a paper’s citations. While it’s great for critical research evaluation, it doesn’t go beyond citation analysis, so it's more limited compared to AI tools for research that can handle summaries or literature reviews. Best for: Evaluating the credibility of papers by analysing how they're cited. Pros: Unique citation context, helpful for systematic reviews and academic writing. Cons: Paid subscription, limited full-paper analysis, interface has a slight learning curve. ChatGPT (with research plugins or file uploads) ChatGPT, when set up with research plugins or file upload access, can act as a flexible research assistant. It can read, summarise, and even suggest related works based on uploaded papers. Still, it relies a lot on the right setup and prompts. Its academic search ability depends on external sources, which can make it a bit clunky next to more focused AI tools for research. Best for: Explaining complex concepts, brainstorming ideas, and drafting academic content in natural language. Pros: Conversational, versatile, supports long-form content creation. Cons: Information generated contains inaccuracies and requires fact-checking. Connected Papers Connected Papers helps you build visual graphs of related papers, making it easier to explore a research area and spark new ideas. It's especially useful for seeing how studies are linked, unlike some AI tools for research. Best for: Finding related research papers using a visual map. Pros: Easy to see how papers are connected and discover important related work. Cons: Doesn’t include all journals; limited to discovery, not for deep research. AI-driven Literature Review Tools Ah, yes, the time-loop of literature review, but in 2025, AI steps in like a compass guiding you through a forest of ideas. Now you can save weeks, if not months, just by using one of these AI-driven literature review tools below. Zendy As one of the most comprehensive AI tool for research, Zendy combines content discovery with artificial intelligence features to support your entire literature review process. It provides researchers with seamless access to millions of open-access as well as paywalled articles from top peer-reviewed journals—all in one place. That means less time switching between databases and more time actually engaging with the research. Best for: Discovering, reading, and organising research papers to build your literature review. Pros: Access to 40 M+ peer-reviewed papers, advanced search capabilities, and integrated AI tools like ZAIA for reference-backed answers, summarisation, and key phrase extraction. Cons: Some AI features require a paid subscription. Litmaps Litmaps helps you visually map the connections between research papers, making it easier to trace how studies are related and how knowledge of a topic has evolved over time. Researchers can start with a single ‘key’ paper, and Litmaps will generate an interactive citation network, revealing clusters of related research. This kind of visual exploration sets it apart from other AI tools for research. Best for: Tracking and creating citation network charts to understand the development of a research field. Pros: Discover new papers through citation mapping, identify research trends, and organising your reading in a dynamic timeline. Cons: Does not summarise or analyse paper content. ResearchPal ResearchPal is an AI-powered assistant designed to support researchers with literature reviews, reference management, and content refinement. It integrates smoothly with popular tools like Zotero and Mendeley, making it easy to organise sources and streamline your writing workflow. With features like article summarisation and citation generation, it can significantly speed up the research process, though it's still important to review outputs for accuracy and comprehensiveness. Best for: Automating and accelerating the literature review process. Pros: Quickly generates literature reviews with citations; integrates with reference managers. Cons: Full access to long-form review generation is limited to paid plans. Sourcely Sourcely scans a vast database of over 200 million academic papers to find credible sources, create citations, and in many cases, access full-text PDFs for free. It’s handy for quickly pulling together a bibliography. However, it focuses solely on source discovery and citation, without additional features like summarisation or visual data mapping. Best for: Discovering and citing credible academic sources for essays and research projects. Pros: Allows you to paste your writing and receive source suggestions with ready-to-use citations. Cons: May exclude papers behind paywalls, limiting access to certain publications. Consensus Consensus is an AI-powered academic search engine that extracts insights directly from a large database of peer-reviewed papers. As its name suggests, it highlights how much consensus exists among researchers on a given topic, offering a quick, evidence-based snapshot of scientific agreement. Ideal for fact-checking or validating claims, it presents clear yes/no answers with citation links. However, its deeper features are gated behind a paid plan. Best for: Fast fact-checking and valuable insight into the prevailing intellectual currents and general dispositions within academic circles. Pros: Draws from peer-reviewed sources; provides concise answers with source links; user-friendly interface. Cons: Focuses mostly on scientific topics; doesn’t analyse full papers; offers minimal contextual depth in free version. R Discovery R Discovery is a mobile-first app designed to help you stay on top of research that matches your interests. It delivers a personalised feed of academic papers, along with unique features like audio versions, PDF chat, and language translations, making it ideal for researchers who prefer a flexible, on-the-go experience. While great for discovery, it’s not built for deep analysis or summarisation. Best for: Staying updated with new research papers tailored to your interests. Pros: Personalised feed, daily research digests, audio and translation options for added accessibility. Cons: Lacks advanced summarisation or analytical tools; best suited for discovery rather than in-depth research. Scinapse.io Scinapse is a free academic search tool that focuses on showing you papers based on real citation networks. It avoids hallucinations by sticking to verified sources. While it’s reliable for finding accurate results, it lacks some of the features you’d expect from a full-service AI tools for research. Best for: Basic paper search functionality which is available for free. Pros: Provides AI-generated mini-reviews and trend analyses within specific research fields, saving time on literature reviews. Cons: Limited full-text access. AI-powered Writing Assistants A good research article or study is recognised by how it’s written. It’s simple: you can’t present your findings effectively without knowing how to communicate them accurately through your writing. Below, you’ll find top AI tools for research to improve your academic writing skills. PaperPal PaperPal is an AI writing assistant designed to enhance academic writing by improving grammar, clarity, and tone—particularly in formal research papers. It’s ideal for refining your drafts and ensuring your language meets scholarly standards. While it doesn't assist with sourcing or literature review, it excels at polishing what you've already written. Best for: Citation assistance and plagiarism detection. Pros: Seamless integration with MS Word, Google Docs, and Overleaf. Cons: Limited utility for non-academic or creative writing tasks. Jenny.AI Jenny.AI is built for speed. You give it a prompt or topic, and it drafts content for different sections of your paper. Also, It’s helpful for getting a rough version down quickly, but the writing usually needs to be checked and edited. While it's great for producing rough drafts, the output typically requires careful review and editing. Compared to any other AI tools for research, Jenny.AI is better suited for writing assistance than for deep research or critical analysis. Best for: Generating academic content drafts and accelerating the writing process. Pros: Includes features like AI autocomplete and section-by-section writing support. Cons: The line-by-line content generation approach may slow down the writing process for bulk content creation. Aithor Aithor is like a blank page with backup. You can write directly in it, ask for content suggestions, or get help rewording things. It’s useful when you’re stuck or unsure how to start, though you’ll still need to tweak and fact-check what it gives you. Best for: Generating structured academic drafts with AI assistance. Pros: Supports multiple languages and allows customisation of tone and style. Cons: User reviews indicate dissatisfaction with output quality and pricing. Wisio.app Wisio is more like a writing coach. You upload your academic draft and receive detailed feedback—either from AI or real human editors, specifically tailored to scientific and scholarly writing. It’s not designed for fast writing or research help, it’s a powerful tool for refining your work once the core writing is complete, especially if you’ve already used AI tools for research earlier in the process. Best for: Refining, editing, and translating academic manuscripts with AI-assisted or human editorial support. Pros: Offers grammar, style, and clarity suggestions tailored to scientific writing; supports multilingual editing. Cons: AI-generated feedback still requires human oversight to ensure accuracy and contextual appropriateness. Trinka AI One of its most helpful features is how it spots citation inconsistencies, those little things that can trip you up in academic writing. Additionally, it helps with your whole writing process and figures out the right citation style and applies it automatically. Trinka AI works with a wide range of sources and even helps with your writing quality. Best for: Academic and technical writing, including research papers, theses, and reports. Pros: Contextual spelling and punctuation checks. Cons: Limited support for languages other than English. Grammarly Grammarly helps researchers write more clearly, accurately, and professionally by correcting grammar, punctuation, and spelling errors in real time. It also improves clarity and tone, making academic writing more precise and formal—especially useful for non-native English speakers. With features like a plagiarism checker and integration with tools like Word and Google Docs, Grammarly saves time and ensures research papers, proposals, and emails are polished and publication-ready. Best for: Academic grammar and clarity. Pros: Real-time feedback, browser integration. Cons: Limited research-specific guidance. AI Tools for Data Analysis in Research Some tools focus on cleaning and organising your data, while others assist with analysis or even visualising results. Whatever stage of data analysis you’re at, there are plenty of AI tools for research that can make data analysis quicker and a lot less frustrating, that can save you time and help you focus on what the data is actually saying. Julius AI Julius AI is designed for quick, conversational data analysis. You upload a spreadsheet or dataset, and it answers questions in plain language, no code or formulas needed. It’s one the AI tools for research that feels more like a chat than a technical task. It’s useful when you want insights fast without getting deep into stats or software. Best for: Performing statistical analyses. Pros: Offers statistical tests, regression analysis, and forecasting models. Cons: Free accounts may face limitations in handling large datasets. Vizly Vizly turns spreadsheets into visual summaries. It focuses on data storytelling, charts, trends, and patterns, and explains what they mean. You don’t need technical skills to use it, which makes it great for researchers who want clean visuals and summaries without fiddling with design tools. Best for: Quickly analysing and visualising data using AI. Pros: Offers features like data cleaning, statistical analysis, and machine learning. Cons: Free tier limits users to 10 AI interactions per month. ChatGPT-4o ChatGPT-4o can work with uploaded datasets and help analyse, clean, or explain the data. While it’s not a dedicated data tool, its flexibility makes it handy for quick calculations, summaries, and exploring ideas. The quality of results depends on how clearly you phrase your question, it’s a flexible addition to your set of AI tools for research. Best for: Generating reports, and answer questions about datasets. Pros: Useful for brainstorming and troubleshooting data analysis problems. Cons: Doesn’t directly process raw data files. Polymer Polymer is a no-code AI tool that transforms your data into interactive dashboards with minimal setup. Simply upload a file, and Polymer automatically generates searchable tables, charts, and filters, making it ideal for uncovering patterns, presenting insights, and collaborating with teams. It’s especially useful for researchers looking to turn complex data into clear, shareable visuals without needing technical skills. Best for: Simplifying data analysis through AI-generated dashboards and visualisations. Pros: Easy to use; enables effortless collaboration and dashboard sharing with team members. Cons: Limited template variety may restrict visual customisation. Qlik Qlik is a more advanced tool used in both business and research. It’s strong in connecting large datasets, analysing them from multiple angles, and visualising results. It does have a learning curve, but it’s powerful if you’re working with a lot of complex data. Best for: Data integration from many sources. Pros: Easy drag-and-drop interface for building visual reports. Cons: Requires setup and training to use effectively. AI Paraphrasing Tools for Students These tools help rephrase sentences in a clear and more natural way. They’re especially useful when you’re stuck figuring out how to say something differently or want to check if your version still makes sense. Just remember, it’s still important to understand what you're writing and not rely on the tool to do all the thinking. But keep in mind that paraphrasing doesn't avoid plagiarism, and you still need to cite sources. Here are some of the best AI tools for research that focus on paraphrasing: Ref-n-write It is a must-have AI-powered writing assistant for researchers, available as a plugin for Microsoft Word, Google Docs, and other Office-compatible platforms.Ref-n-write helps you rephrase academic sentences while maintaining formal tone and consistency, making it especially valuable for non-native English speakers and those working on research-intensive documents. It also includes a comprehensive academic phrasebank and example sentences for common academic topics, It is considered one of the suitable AI tools for research, particularly for writing, editing, and proofreading Best for: Rewriting and paraphrasing academic text to improve clarity. Pros: Offers an extensive academic phrasebank with discipline-specific expressions. Cons: Rephrasing suggestions may occasionally feel repetitive or formulaic. SciSpace SciSpace isn’t just for paraphrasing, it’s a broader AI tool that helps you understand and rewrite academic content. By uploading a PDF, you can ask questions, receive simplified explanations, or rephrase selected sections of the text. This makes it particularly helpful for grasping difficult material and improving how you articulate academic concepts in your own words. Best for: Clarifying and rephrasing academic content to meet scholarly writing standards. Pros: Intuitive interface with a minimal learning curve; excellent for breaking down complex texts. Cons: Paraphrasing is confined to the text editor; full-document upload and processing are not supported. MyEssayWriter.ai Just enter your topic, academic level, and essay type. MyEssayWriter.ai is a more casual AI writing tool that helps you paraphrase or generate essays based on short prompts. It’s quick and easy to use, but the writing can feel generic. It’s better suited for brainstorming or first drafts rather than polished academic work. Best for: Rephrasing academic content while preserving meaning, ideal for early-stage drafting. Pros: Supports paraphrasing in over 27 languages. Cons: May require multiple inputs to refine output; not suited for high-level academic writing without further editing. Scribbr Scribbr is designed to enhance academic writing quality and maintain integrity. Its core features focus on using AI to detect plagiarism, provide proofreading, citation assistance, and AI content identification, which makes it a great option for you if you are looking to clean up your writing without changing the meaning. Whether you're preparing essays, theses, or academic reports, Scribbr helps ensure your academic writing is polished and properly cited. Best for: Students seeking to improve clarity and ensure academic accuracy in their writing. Pros: Allows you to click on words to view and select appropriate synonyms for improved phrasing. Cons: Input is limited to 125 words per submission, which may restrict larger edits. Rewrite Guru Rewrite Guru helps you rephrase text with varying levels of creativity and structural change. Whether you want a simple rewording or a more inventive rewrite, the tool gives you control over how much the sentence is altered. It's easy to use, but less focused on academic tone, so the results may need adjusting for formal assignments. Best for: Rewriting sentences or paragraphs with customisable rephrasing depth. Pros: Offers built-in grammar and plagiarism checkers. Cons: Some users experience slower processing speed and occasional inconsistencies in output quality. AI Productivity Tools for Researchers True accessibility means being able to access, use, and benefit from a tool with ease. In research, that also means saving time. The following five AI tools streamline key academic tasks—whether you're taking notes, managing research, organising tasks, writing, or presenting—so you can focus more on complex thought and analyses and less on switching between platforms. Otter AI Otter AI turns your lectures, voice notes, or group chats into written transcripts. As one of the effective AI tools for research, it’s especially handy during class so you can focus on listening without worrying about taking notes. Best for: Recording and transcribing lectures. Pros: Converts spoken words into text in real-time, eliminating manual note-taking. Cons: Transcription accuracy may vary depending on audio quality and speaker accents. Bit.ai Bit.ai is a workspace where you can bring your research, notes, links, and files together in one document. It’s especially useful for managing complex projects or working in teams, as it supports real-time collaboration and keeps everything organised in one place. Whether you're preparing a thesis, coordinating a group assignment, or compiling research sources, Bit.ai helps streamline your workflow. Best for: Organising academic materials and collaborating on research documents. Pros: Offers 70+ templates tailored for academic needs, including research papers and thesis documents. Cons: AI-powered features are only available on paid plans. Todoist Todoist is a powerful task management tool that helps researchers break down complex projects, like writing a paper, conducting experiments, or preparing grant applications, into clear, manageable steps. You can set deadlines, assign priorities, track progress, and collaborate with co-authors by sharing tasks and leaving comments. It’s a great way to stay organised and focused throughout your academic workflow. Best for: Structuring and managing academic projects, deadlines, and study schedules. Pros: Allows you to create separate projects for courses, papers, and assignments with prioritised task tracking. Cons: May feel feature-heavy for users with very simple task management needs. Notion Notion is an all-in-one workspace that helps researchers organise their work, from notes and papers to project timelines and references. Its flexible database structure allows you to categorise, tag, and filter content so you can easily retrieve what you need. With the ability to build custom dashboards, track deadlines, and collaborate with peers, Notion is a powerful tool for managing every stage of the research process in one central place. Best for: Organising research notes, managing projects, and collaborating with peers in one workspace. Pros: Combines notes, tasks, databases, and calendars to streamline your research process. Cons: Full functionality depends on internet connection; offline capabilities are restricted. AI Tools for Thesis Writing Writing a thesis takes time, focus, and a lot of patience. Between gathering sources, keeping your notes in order, and turning complex ideas into a clear, well-structured argument, the process can feel overwhelming. That’s where a practical AI tool for research can make a real difference. These tools won’t write your thesis for you, but they can help you stay organised, improve your writing, and work more efficiently. ThesisAI ThesisAI is an advanced AI writing assistant that guides you through the thesis writing process with just a single prompt. It can generate up to 50 pages, include inline citations and reference up to 100 reference papers. You can also rewrite specific sections to improve clarity or tone. With support for over 20 languages and compatibility with formats like PDF, Word, LaTeX, and BibTeX, ThesisAI is one of the most versatile tools for large-scale research projects. Best for: Generating full-length academic documents with citations. Pros: Offers multilingual support and exports to multiple academic formats including PDF, Word, LaTeX and BibTeX. Cons: Charges per document (starting at $5), which may become costly for frequent users. Gatsbi Gatsbi positions itself as an AI co-scientist. One of its strengths is sourcing. It avoids making things up and sticks to real, verifiable references, just like ZAIA. Gatsbi can help apply research methodologies like TRIZ, draft papers, patents, write-ups, or technical documents with built-in support for equations, citations, figures, and data tables. It’s particularly useful for interdisciplinary work that demands both accuracy and professionalism. Best for: Structuring and formatting academic documents with citations, visuals, and methodical frameworks. Pros: Automates complex formatting tasks while maintaining academic integrity and quality. Cons: Full access requires a paid subscription, which may not be suitable for all budgets. Writefull Writefull is an AI thesis writing assistant designed specifically for academic and scientific tone. It offers tools to help you improve grammar, vocabulary, and overall clarity in your writing. Writefull integrates with platforms like Microsoft Word and Overleaf, providing real-time language feedback within these environments. Additionally, it includes features such as paraphrasing suggestions, abstract and title generators, and LaTeX code assistance for equations and tables. Among the growing field of AI tools for research, Writefull stands out for its focus on scientific accuracy. Best for: Academic writers seeking AI-driven assistance to improve the quality and clarity of their research papers, theses, or scholarly articles. Pros: Assists in creating concise and relevant titles and abstracts tailored to the content of your paper. Cons: The AI may sometimes misinterpret context, especially with technical terms or nuanced scientific content. Thesify Thesify is a web-based platform accessible across devices, designed to support graduate students, PhD candidates, and academic researchers working on essays, theses, dissertations, or journal articles. Unlike generative writing tools, Thesify focuses on enhancing critical thinking and strengthening academic writing through structured feedback. It also supports ethical AI use in academic writing while preserving the researcher’s original way of expressing ideas and insights, serving as a thought partner rather than a content generator. It’s a reminder that even the best AI tool for research should complement your reasoning, not suspend it. Best for: Students and researchers seeking structured, expert-level feedback on academic writing, particularly for essays, theses, and research papers. Pros: Evaluates clarity, logical flow, and evidence use; provides actionable suggestions to strengthen scholarly research questions. Cons: Does not provide grammar or spelling corrections, making it best used alongside tools like Grammarly. AI Citation Management Tools Using AI for citation management is easy, you can upload a paper or type in a reference, and AI takes care of the rest, saving time and reducing the risk of plagiarism and intellectual property violations. Here are the top citation management and referencing tools in 2025 for researchers and students. Zotero Zotero is a free, open-source tool that helps you keep all your research in one place. You can collect, organise, annotate, cite, and share research materials, sort them into folders, and tag them however you like. It works with Word, LibreOffice, and Google Docs, so you can add citations and build your bibliography as you write. You can also highlight and make notes on PDFs, share your library with others, and sync everything across your devices. It's a solid option if you want something flexible and collaborative without paying a subscription fee. Best for: Collaborative reference management with no usage restrictions. Pros: Free, open-source citation management; supports thousands of citation styles (APA, MLA, Chicago, etc.); enables real-time library sharing and syncing. Cons: Free storage is limited; expanded cloud storage requires a paid upgrade. EndNote EndNote is a paid reference manager that’s popular with researchers handling big projects. It helps you gather and organise references, pull in full-text articles, and create citations in thousands of styles. Its “Cite While You Write” feature lets you add references as you go, and it updates them automatically when things change. You can also annotate PDFs and sync your work across desktop, iPad, and online accounts. Best for: Complex writing and research workflows. Pros: Integrates well with Word for easy citation insertion. Cons: Can be complex and overwhelming for new users. Mendeley Mendeley is a free reference manager combined with an academic social network, You can organise references, annotate PDFs, generate citations, collaborate with other researchers online by joining related groups, and discover new papers based on what you’re already reading. It’s a good tool that blends reference management with a built-in academic social network for collaboration and discovery. It still works well alongside more focused AI tool for research. Best for: Connecting with other researchers. Pros: Free to use with good PDF management. Cons: Interface can be a bit clunky sometimes. RefWorks RefWorks is a cloud-based reference manager designed for students, researchers, and academic institutions. You can store references, organise them into folders, and generate citations in different styles. It’s often used by universities and works best when integrated with library databases. Best for: Managing references and creating citations. Pros: Good for group projects with collaborative features. Cons: Mostly available via institutional subscription. AI Tools for Creating Research Presentations Presenting your research effectively is just as important as conducting it. Slides help your audience stay engaged by highlighting key points, visuals, and summaries, making complex ideas easier to follow. This is especially valuable at conferences or during thesis defenses, or classroom presentations, where time is limited and clarity is crucial. Well-structured presentations also encourage questions and feedback, a vital part of the academic process. Here are top AI tools for research presentations that can save you time while helping deliver your findings in a polished, professional format. Gamma A personal favourite for many, Gamma is considered one of the best AI tools for research presentations; easy to use, fast, and the UI is just as clean as it should be. It's best for those seeking to produce polished content efficiently. By leveraging advanced AI capabilities, Gamma enables users to generate visually appealing materials from simple text prompts, significantly reducing the time and effort typically required for design and formatting Best for: Quickly converting academic content into engaging, well-structured presentations. Pros: Generates clean, structured slides from text inputs like research papers and outlines in minutes. Cons: Output may require manual editing to improve accuracy, context, or visual impact. Presentations.AI Create your free account, upload your research, and voilà— you're good to go! According to many, it is the simplest AI tool to turn your research paper into a few stunning slides. Plus, Presentations.AI supports real-time collaboration, so teams can work together, leave feedback, and track tasks all within the app. Best for: Researchers who want to transform complex academic ideas into clear, professionally designed slides. Pros: Supports multiple languages and real-time collaboration, making it accessible for a global audience. Cons: Customisation options may be limited for users seeking highly specific design layouts. PopAI Another great AI tool that simplifies the process of turning research findings into visually engaging slides, no design experience needed. PopAI offers a range of visual formats, including charts, infographics, and illustrations, making your content more accessible and impactful. Ideal for academic settings, PopAI enables easy export to use for conference talks, class presentations, or thesis defenses. Best for: Creating interactive teaching materials with embedded quizzes and multimedia. Pros: Built-in AI writing assistant, paraphraser, and image/video generator. Cons: The wide array of features may require a learning curve to use effectively. AiPPT AiPPT is useful for professionals, educators, and students who want to save time on slide design and focus on their message. Key features include one-click document-to-slide conversion, a large template library, smart adaptation of your content to fit slides, and real-time collaboration so multiple people can work on the same presentation together. AiPPT also lets you embed videos, polls, and interactive charts to make your slides more engaging Best for: Converting essays, assignments, and lecture notes into structured presentations. Pros: AI intelligently formats text, images, and data to fit slides aesthetically and contextually. Cons: While templates are robust, advanced customisation may require manual adjustments. Conclusion AI is no longer just a tool in the research process, it’s a collaborator. However, these tools aren’t perfect; they often vary in accuracy, depth, and usability. For this reason, not every tool will be a good fit for every stage of research. As a result, it’s important to explore, test, and use a multitude of tools that fit your needs. As these technologies continue to evolve, staying curious and adaptable is the best way to keep your research sharp, stay competitive, and be ready for the future. Most importantly, always fact-check your sources, verify references, and critically review AI-generated content for clarity, accuracy, and originality. When using AI for writing or paraphrasing, ensure the final output reflects your own understanding, voice, and academic intent. Don’t forget that Ethical publication practices should always come first. Follow your institution’s policies on AI use, cite AI-generated assistance where necessary, and avoid relying on tools in ways that could be considered plagiarism or lead to misrepresentation. .wp-block-image img { max-width: 85% !important; margin-left: auto !important; margin-right: auto !important; }